During a discussion on industrial analytics at a recent Smart Factory conference in Columbus, Ohio, an IT professional in the audience said that he planned to upload his machine data to online analytical processing (OLAP) cubes and run Business Intelligence (BI) applications on top of the data. The analytics professionals on stage appeared horrified for a moment and went to great lengths to explain the distinction between industrial analytics and BI.
Similarly, companies might question the need for an analytics solution when they already have a BI tool that provides a dashboard for their industrial metrics.
Industrial Analytics is NOT the Same as Business Intelligence
OLAP Cubes and BI Software
OLAP technologies came into existence to solve the data reporting shortcomings of existing relational databases of enterprise systems; specifically, they were created to answer business questions rather than IT questions. Before OLAP, running complex queries that sort through millions or billions of records in normalized tables had become increasingly time-consuming. In fact, some manufacturing reports mention that users started queries when they left the plant in the evening and expected the results to be ready when they returned the next morning. In contrast to this, OLAP stores pre-configured in a multi-dimensional database that enables faster data retrieval than running queries through traditional relational databases. BI software that utilizes OLAP data cubes can aggregate, slice and dice, and group data by different dimensions to provide charts, graphs, and other visualizations much faster than previously used databases and enterprise data warehouses (EDW).
IIoT Analytics Applications
While OLAP solutions enabled faster data retrieval for structured data in data cubes, their strength is also their weakness. That is, they are optimized for structured databases where the queries are known in advance; they are NOT designed for the magnitude and complexity of data available in today’s factories. Manufacturing plants create data in the petabyte-per-day range, and scale upwards from there. Additionally, there are different data formats (e.g., time-series data) that originate from thousands of inexpensive sensors attached to machines, plus data from Edge devices on the shop-floor, enterprise data from servers, the Cloud, and more.
Industrial Internet of Things (IIoT) analytics solutions are designed to handle this deluge of data, empowering manufacturers to analyze and optimize their factory data, combine it with enterprise data, and drive new use cases that were not possible with BI tools and OLAP cubes. With applications that run on Cloud, plant data can be accessed even outside the plant, and Edge analytics allow real-time data processing at the asset level for instantaneous decision making.
While analytics applications can also run primary analysis - slice and dice numbers and produce charts in dashboards - their primary differentiator is identifying patterns and trends in data to analyze failure instances, predict future failures, and prescribe corrective actions. These queries are not generally defined in advance of the data collection and storage, and so the database cannot be optimized for specific questions.
IIoT analytics applications are designed to condition and contextualize massive streams of data and produce intelligible results from that data on the fly. This is significantly more complex than the extract, transform, load (ETL) capabilities of traditional databases that support BI tools. The complexity arises partly due to the massive number of variables, and the requirement for the applications to suggest potential variables of interest to help narrow down the problem space. Therefore, machine learning (ML) and artificial intelligence (AI) are so often part of analytics platforms.
After data ingestion, conditioning, and contextualization, IIoT analytics can be integrated to the continuous stream of data to detect anomalies on the fly, and automatically suggest solutions; this enables operators, engineers, and managers to respond more quickly to issues, and in turn, create more stable and efficient processes.
How ML and AI Are Used
When combining factory data with enterprise data, companies often choose to use ML or AI to address the combination of structured, unstructured, and semi-structured data and draw insights from this rapidly changing dataset. These same ML/AI technologies often provide the foundation for predictive or prescriptive analytics for the shop floor.
Takeaways
While BI tools can provide business answers to business questions, IIoT analytics with predictive and prescriptive analytics can provide answers to newer questions companies didn’t know to ask in the first place. However, that doesn’t mean IIoT analytics are going to wipe out existing enterprise data warehouses and BI applications. Manufacturers who already have a BI tool can still benefit greatly investing in IIoT analytics applications.
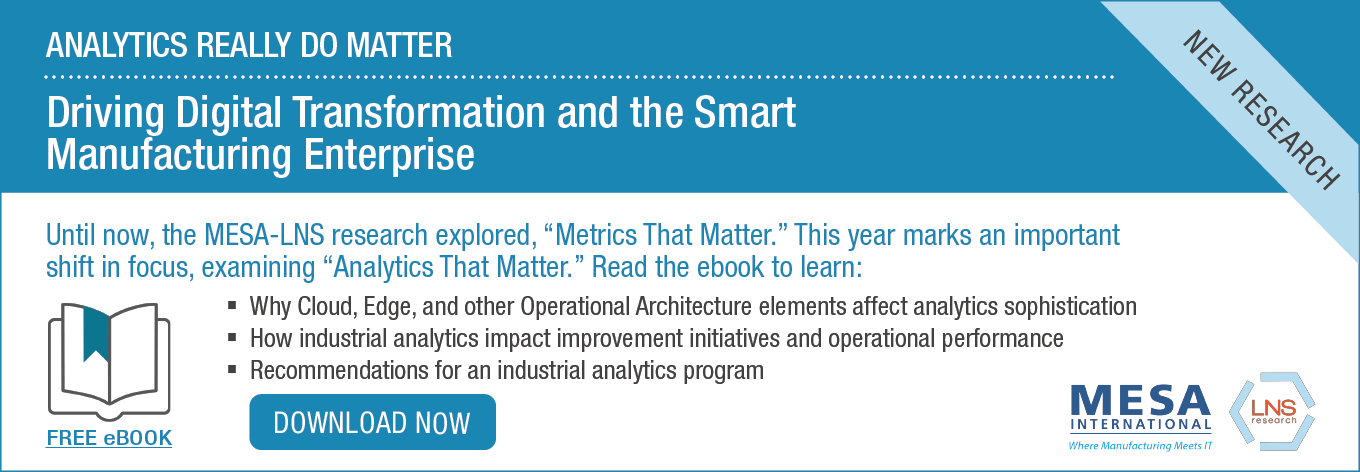