As regular readers of the LNS Research blog are aware, we are big proponents of using metrics. Our Metrics That Matter research done in conjunction with MESA highlighted what metrics manufacturers are using to manage their operations. Asset Performance Management (APM), like Manufacturing Operations Management (MOM), also has a rich set of metrics with twenty or more commonly used today.
With so many choices it should be a simple matter of just choosing the ones that best fit your business right? Unfortunately, it isn’t so easy. Choosing the wrong metrics can be worse than not using metrics at all.
The Right APM Metrics and KPIs are Role-Specific
The production manager has a different view of APM than a safety director or a purchasing manager.
The production manager is generally concerned with plant productivity and focuses on APM metrics that provide insight on asset reliability and availability. A purchasing manager is most likely concerned with material costs, compliance to contracts, and minimizing shipping expenses. A safety director is focused on hazardous materials management, process safety failures related to equipment, and safety procedure compliance. Of course, the higher up the organizational chart you go, the more the interest becomes financial and broader and more abstract.
The other aspect of this is that the time span and scope of the metrics vary by role. A first line supervisor is going to be interested in metrics that are calculated in real time and indicate problems that are occurring now. Maintenance supervisors have both a need for metrics that reflect immediate problems as well as historical metrics that show performance over time. Senior managers at the corporate level are typically looking at figures that roll up asset information over the course of days, weeks, or months and across multiple locations.
So, it is clear no simple set of five or ten metrics will satisfy everyone. We’ll look at some maintenance and production metrics next and then some health and safety, procurement, quality, and energy-related APM metrics in future posts.
Metrics That Gauge Maintenance Performance
Plant engineers and maintenance staff are the groups typically most responsible for the execution of an asset performance management program. They typically have needs that are both shorter and longer-term. On a day-to-day basis a few of the metrics often monitored are:
- Work Order Closure Rate: This reflects the number of maintenance work orders actually closed as a percentage of those planned to be completed. It is indicative of either planning efficacy or labor efficiency. It is important not to let outlier events trigger unwarranted concern as the metric can vary widely in those situations.
- Schedule Compliance: Closely related to closure rate is schedule compliance. This is a measure of adherence to the planned work schedule. It can be negatively impacted by unscheduled work as well as poorly planned work, or a number of other factors.
- Percent Productive Time: This is a measure of actual maintenance work as a percentage of total scheduled maintenance labor time. It can be difficult to correctly gather the data required for an accurate calculation but it is highly indicative of the health of the maintenance organization and the efficiency of the maintenance processes.
To understand longer-term performance of the maintenance organization, some of the longer-term metrics used are:
- Mean Time Between Failures: A classic metric that measures the time between failures on equipment. The difficulty with this metric is it may reflect equipment design issues or suitability for the process as much as it reflects maintenance performance.
- Percent Emergency Work: Measure of the percent of unscheduled versus total work. This is an indicator of the effectiveness of PM and PdM programs.
- Percent Planned Work: This is essentially the inverse of the above.
- Planned/Unplanned Ratio: A variation on the above two metrics.
- Percent Overtime: This is a labor force size metric. If it's too high, it may reflect insufficient or unskilled maintenance labor. If it's extremely low, it may signal surplus labor negatively impacting maintenance cost per unit production.
- Maintenance Labor Cost as a Percent of Total Maintenance Cost: An indicator of labor force efficiency and effectiveness. It can also be impacted by poor procurement practices, high contractor use, or other related factors providing a false indicator of performance.
- Contractor Cost as a Percent of Total Maintenance Cost: An indicator of efficient labor force sizing. It can be misleading in turn-around or shut down periods. May be intentionally high due to labor policy issues.
- Labor per Planner: Coupled with planner backlog, this is an indicator of maintenance process efficiency. It is a good indicator of PM/PdM program timeliness and effectiveness.
- Work Order Backlog: This is another indicator of overall maintenance department operations.
Choosing which of these metrics to monitor is really dependent on the current situation at the facility. If an organization only has a single planner and Planner Backlog is not unreasonable, then Labor per Planner likely remains highly static and not indicative of much more than the size of the maintenance department. If the plant only uses contractors for shut down/turn around events, monitoring Contractor Cost percent on a regular basis is wasted effort.
Higher-level Productivity Metrics that are APM-Related
Plant production staff and senior management may be interested in some of the metrics that the maintenance staff uses but more likely is focused on those with a productivity or financial flavor. Some of the useful metrics that senior management and plant production staff typically use are:
- OEE: A measure of availability, quality, and performance. This is a widely known metric and we've written plenty about measuring it and how it should be used.
- Availability: A component of the OEE calculation, Availability is calculated as the fraction of Operating Time divided by Planned Production Time. Besides its role in the OEE calculation, Availability itself is a good indicator of machine health but only if breakage is the primary cause of the downtime. If the downtime is related to production issues, then Availability may not be the best indicator of machinery health.
- Percent Uptime (or downtime): Closely related to Availability is Uptime or the related Downtime (100% minus Uptime percent). It is important to understand if the downtime calculation you are using as a metric includes non-productive time related to queuing, setup or other operational delays or if it is strictly being measured due to scheduled and unscheduled maintenance activity. If it is all-inclusive, then Uptime is essentially the same as Availability. It is less important how you calculate Uptime/Downtime than ensuring all parties understand how it is being calculated.
- Percent Re-plan Due to Outages: This is calculated by dividing the number of re-plans due to machine failures by the total number of re-plans performed for all reasons including material or labor shortages. Along with percent of re-plans, which is the number of production plans that have to be re-planned divided by the number of original production plans, this can be a good indicator of process issues versus maintenance issues.
- Maintenance Cost per Unit Production: This is calculated by taking maintenance cost and dividing by production volume. The danger is if this metric is calculated at too high a level it may not be indicative of specific maintenance issues. Also, it may vary significantly if there are planned turn-around or shut down situations that drive maintenance costs higher while lowering production volumes. So it can be useful in a steady state where costs can be accurately assigned to specific products but it must be used carefully. When used correctly it can help balance the approaches of spending on PM versus run-to-failure.
- Percent Unscheduled Downtime: This is calculated by taking unscheduled downtime and dividing by total downtime. It is indicative of the effectiveness of preventative or predictive maintenance programs.
As noted above, not all metrics are appropriate for all operations. OEE in a facility that manufacturers extremely low volume but high margin parts may not be very high, but plant profitability might be excellent. In a facility where there is intentional redundancy due to a highly abusive process that is hard on equipment, it may have a fairly high re-plan rate but overall production due to the redundancies may still be acceptable. Understanding your process and what each metric tells you about the process is critical to using the metrics correctly and using the correct metrics.
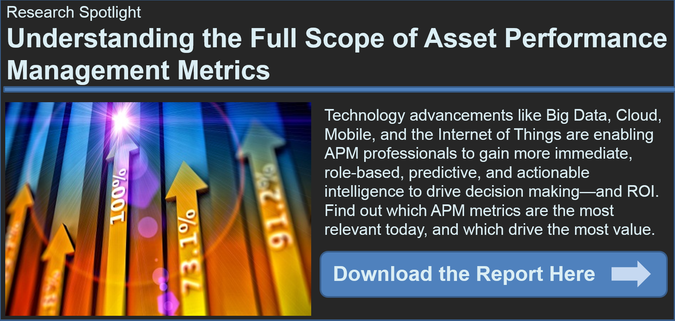