The industrial data and analytics software sector has matured significantly over the last 12-18 months. During this period, the pace of change in technology innovation and customer expectations have both accelerated in tandem, feeding off of each other and propelling the industry forward through a series of milestones—product launches, capital raises, partnership announcements, etc.
This write-up examines three particularly different but complementary software providers—Braincube, Litmus, and Seeq—and their recent milestones in the Industrial Data Platform, Industrial DataOps, and Advanced Industrial Analytics categories, respectively.
Braincube’s Go Beyond Summit Highlights its Focus on Operational Efficiency:
This October, Braincube held its annual Go Beyond Summit in Portland, ME, where it has a sizable presence. The two-day event showcased participation and case studies from several of its customers, including Owens Corning and International Paper.
The event, attended by our very own Matt Littlefield, exhibited Braincube’s Digital Twin, Industrial IoT platform, and advanced analytics capabilities. It also validated Braincube’s strengths, such as its data model replicating material flow across multiple industrial data types, real-time operational dashboards, and vertical industry focus. Braincube also discussed, across use cases, its self-service analytics capabilities, which enable engineers to solve problems without bias. 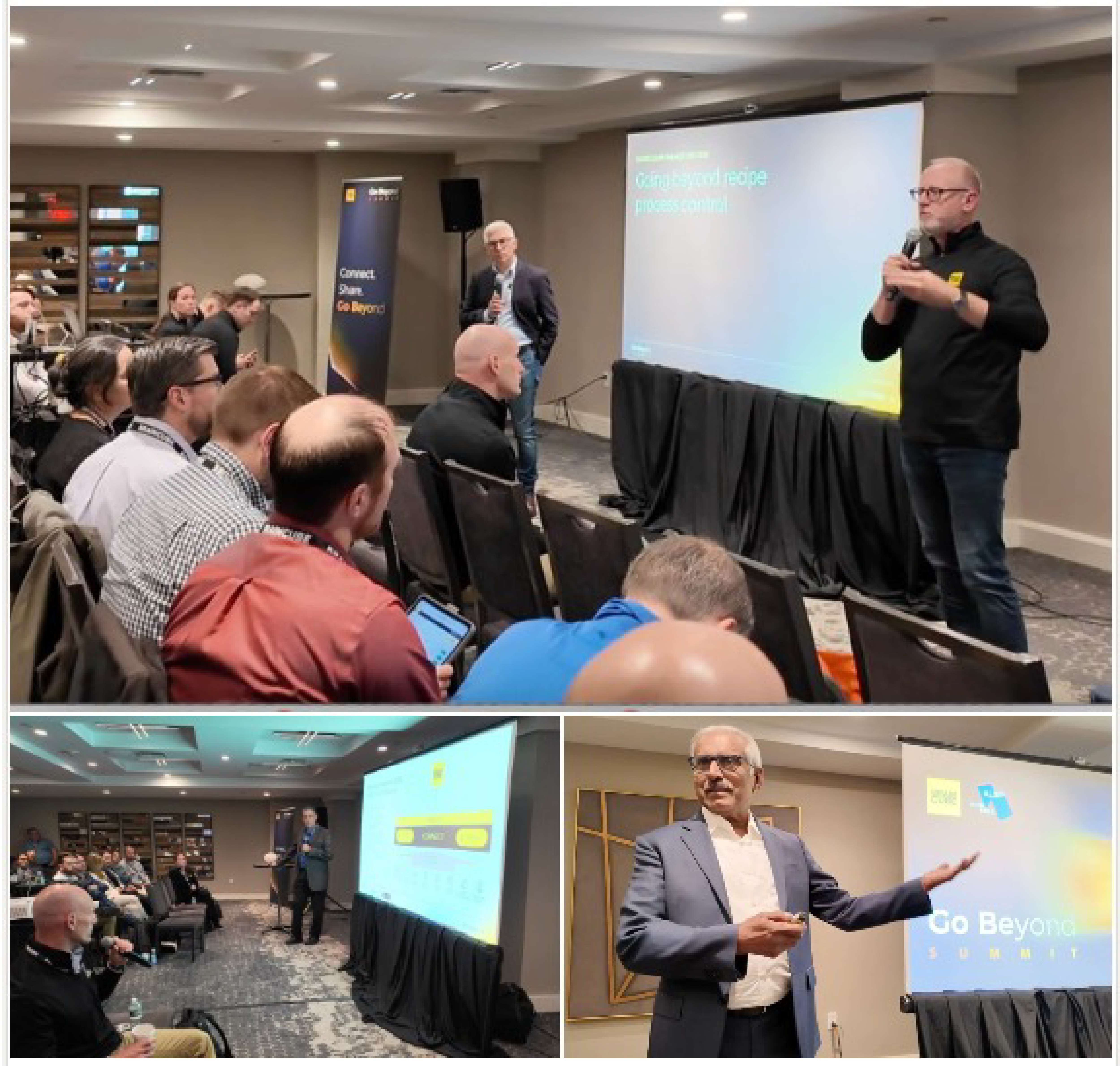
Overall, the Go Beyond summit highlighted Braincube’s data modeling and advanced analytics capabilities and the benefits it has been delivering to its customer base since 2007, when Laurent LaPorte, Hélène Olphe-Galliard, and Sylvain Rubat du Mérac founded the company. Since then, Braincube has grown remarkably, with footprints across 250+ production sites worldwide. Notably, in November 2023, the company completed a Series B round of $91 million in growth equity investment, led by Scottish Equity Partners (SEP) and Bpifrance.
Braincube’s Industrial IoT platform, customizable BI and dashboarding tools, powerful self-service analytics, and proprietary CrossRank AI models are valuable for operational personnel to get actionable insights with relatively less effort and within short periods of time. More recently, focusing on gathering plant-level buy-in and translating operational efficiencies to financial benefits has helped Braincube find itself playing a critical role in reducing waste and improving productivity at scale in several customers’ sites.
LNS Research expects Braincube to continue along its growth journey as it adds more out-of-the-box applications, target user personas, and embedded Industrial AI to its suite of solutions.
Litmus Continues to Push Boundaries of Industrial DataOps:
On the Industrial DataOps side of the fence, Litmus seems to have taken Mark Zuckerberg’s “Move Fast and Break Things” statement to heart. While many early-stage startups in the industrial space have been moving fast, only a select few have set themselves apart with disruptive products that challenge the status quo, redefine industry standards, and truly push the industry forward.
One of the pioneers of Industrial DataOps, Litmus has constantly put solving critical problems for the industry at the forefront of its product development strategy. For instance, the company wrote its own drivers for most of the PLCs in use today as part of Litmus Edge to address problems in establishing seamless connectivity to messy factory floor systems. More recently, they launched their own enterprise-grade MQTT broker to solve data latency and transmission issues.
Finally, in early July, they launched a new product—Litmus UNS—that provides data modeling and standardization on top of their MQTT broker. The unified namespace-based product is focused on solving some of the prevalent problems in MQTT topic structures, querying and metadata, and governance issues such as connecting and managing multiple brokers.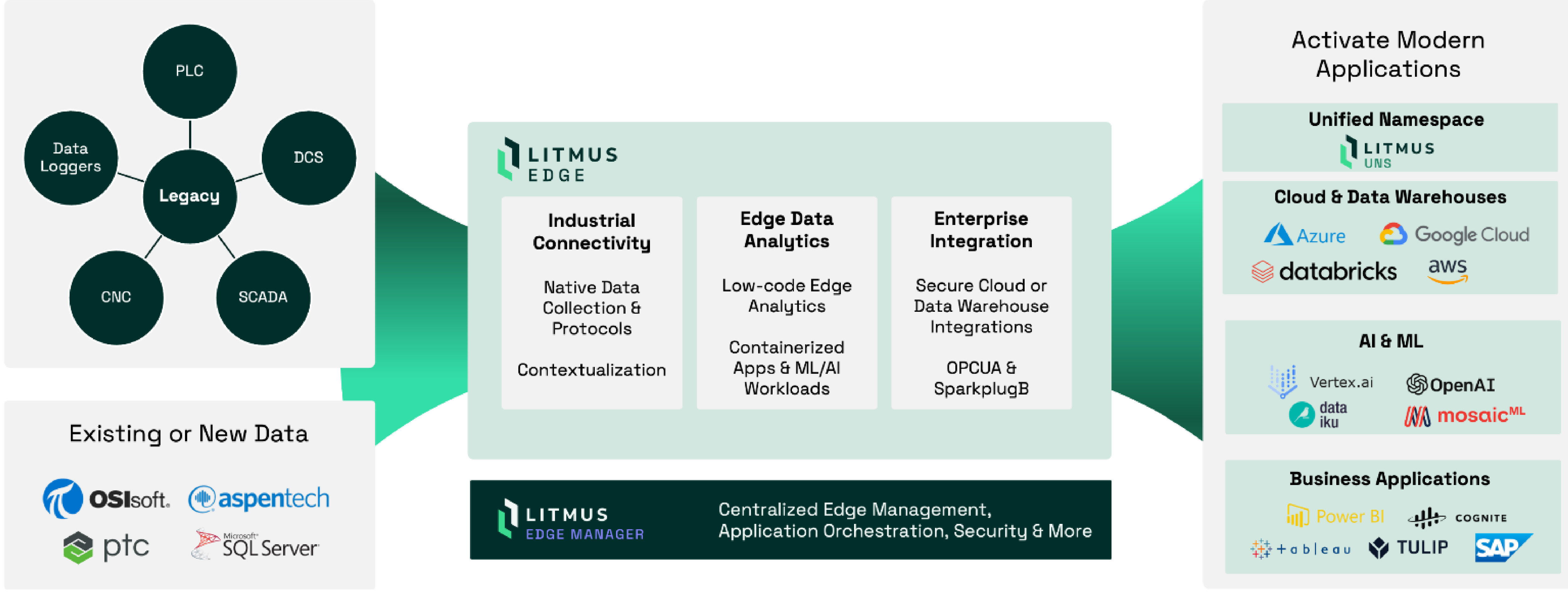
Driven by a bold vision by its co-founders, Vatsal Shah, John Younes, and Sacha Sawaya, Litmus is on a mission to disrupt industrial automation, data connectivity, and contextualization for the better. Headquartered in Santa Clara, California, the company has experienced robust growth, with over 170% year-over-year revenue increase over the last year.
While Litmus’ perseverance in solving problems and pushing boundaries is excellent news for the company and its customers, it also serves as a leading indicator of progress in a broader sense. I am excited about the doors it opens up for the Industrial DataOps space in general, which is becoming increasingly competitive and commoditized. As companies like Litmus continue to push boundaries and move the industry forward, more and more manufacturers will be able to produce more value from their OT data, which is essential for their grand schemes such as Factory of the Future, Autonomous Operations, and Industrial AI initiatives.
Seeq Closes $50 Million in Series D Raise:
In other news, Seeq completed a series D funding round led by Sixth Street Growth, with participation from a few of its existing investors. The $50 million sits atop Seeq’s existing capital raised from Insight Partners, Altira Group, Second Avenue Partners, and Saudi Aramco Energy Ventures, bringing the total capital raised to $165 million. The round was closed in early August, with the announcement that Nari Ansari, Managing Director at Sixth Street, will join Seeq’s Board of Directors.
Interestingly, LNS Research played a small but important role in this process, providing market sizing and advisory services to the Sixth Street team, including Ansari, Chris Perron, and Claire Zhang. In addition to Seeq, the firm has an impressive portfolio, including a series A investment in SaaS-based EQMS and MES provider MasterControl in late 2022.
The investment stands as a testament to Seeq’s strong position and market leadership in the Advanced Industrial Analytics space. Over the last 11 years, the company has built a wealth of connectors and robust self-service analytics for time-series data, making it the preferred solution for process engineers across several continuous and hybrid manufacturing environments. Like Litmus and some other leading companies, Seeq’s customer responsiveness, a key component of its product roadmap strategy, has been essential to its success.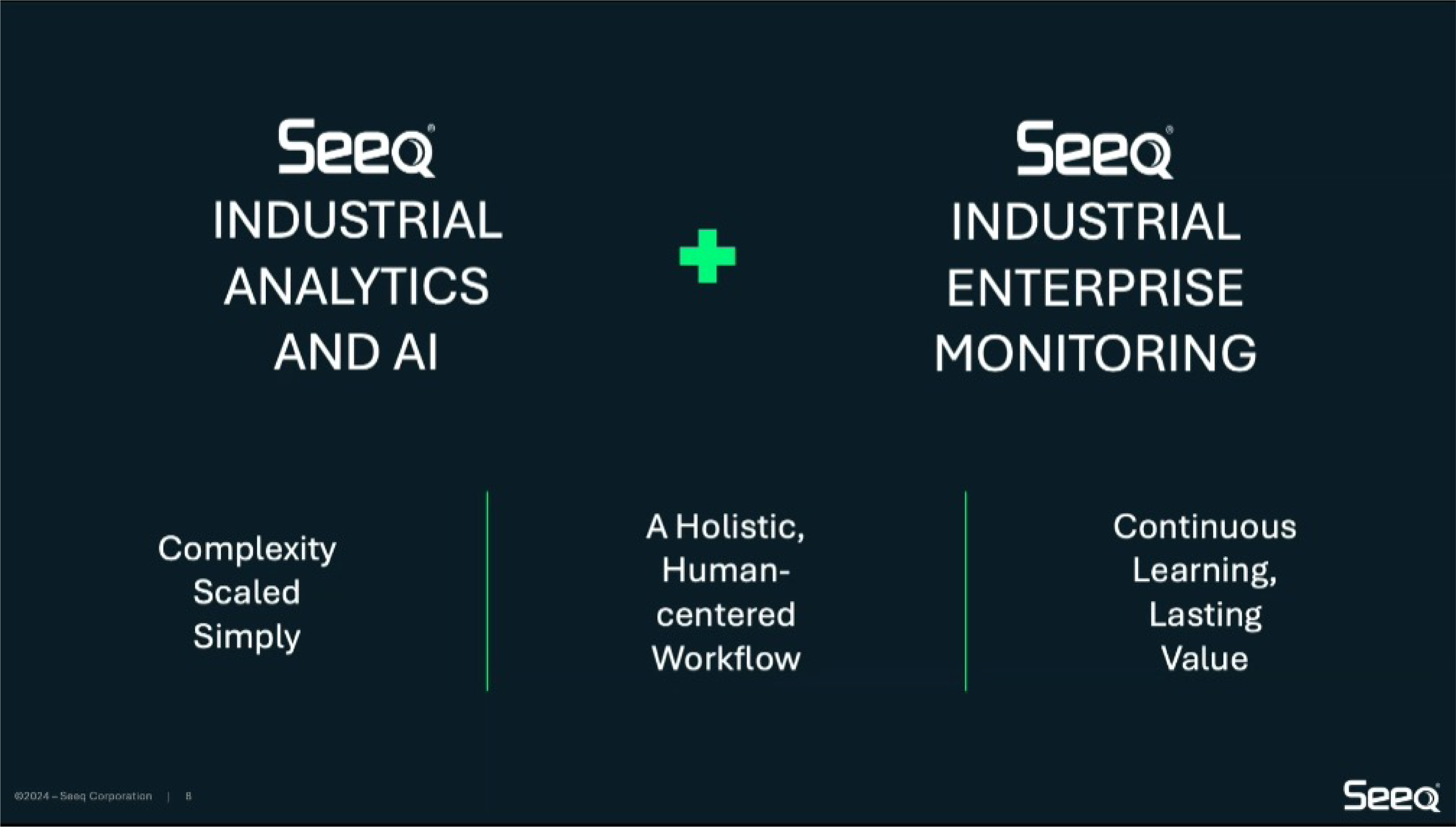
In the recent past, the company has also placed bets to expand its presence in the industrial space further. To begin with, it expanded the scope of its connectors and analytics beyond just time-series data, putting process parameters in context to other transactional data. Additionally, it launched an enterprise-level asset monitoring product aimed at maintenance and reliability engineers. Finally, it also released the Seeq AI Assistant. This generative AI-based product is not just a generic wrap-around ChatGPT but a robust tool to assist users with data analysis, programming, visualization, and problem-solving.
These strategic moves suggest that Seeq is at a crucial inflection point. It aims to grow beyond time-series data and the process engineer user base, checking more boxes in the LNS Research's definition of Advanced Industrial Analytics. Considering the recent updates, the capital infusion, and Seeq’s stellar track record, LNS Research believes it is well-positioned to do so.
Summary & Recommendations:
The last few months have been quite active for the industrial data and analytics space, with announcements like this that continue to reshape the industry and competitive landscape. This heightened activity has resulted in market maturity and growing end-user adoption as manufacturers recognize the potential treasure troves of data stuck within their factory floors.
However, for vendors, this activity level translates to increased customer expectations and competition as some parts of this ecosystem, such as connectivity, become increasingly commoditized. To thrive in such a market, vendors need to rely on more than just organic product growth; they need the right messaging for the right personas, multi-faceted go-to-market approaches, and strategic partnerships and alliances. With every vendor only providing solutions to pieces of the puzzle, all of these focus areas are necessary to maintain and expand market share.
Here are some closing recommendations for the industrial end users as they try to navigate this dynamic technology landscape:
-
-
-
Industrial DataOps is essential, regardless of your approach to architecture. Build vs. Buy is often a highly contentious decision many industrial companies wrestle with. Regardless of your path, leveraging commercially available Industrial DataOps software can save substantial amounts of time and effort to establish seamless edge-to-cloud connectivity, build a layer of abstraction, create a data model, and enable contextualization across multiple levels.
-
An ideal Industrial Data Platform is the cornerstone for a Best-of-Breed approach. At this point, the market is flooded with data and application platforms, each claiming to be an all-encompassing solution but often prioritizing solutions within its ecosystem, even if it ends up with more point solutions and custom integrations.
An ideal Industrial Data Platform must offer more than just internal capabilities. It should offer strong Industrial Data Operations capabilities (or integrate with other commercially available Industrial Data Operations software), enable the creation of a comprehensive enterprise-wide data model, and provide robust applications. Most of all, it needs to provide connectivity and enable open interoperability with external systems, acting as the cornerstone of the operational architecture.
-
Focus on the user persona to ensure scalability. Most industrial data and analytics initiatives begin with identifying business problems and mapping use cases. While that is a good approach, beginning only with the use case without considering how it impacts different user personas creates organizational imbalance and inhibits scaling the initiative over time. Instead, start by identifying user personas that will be leveraging the analytics, what their individual goals, objectives, and priorities are, and then map use cases.
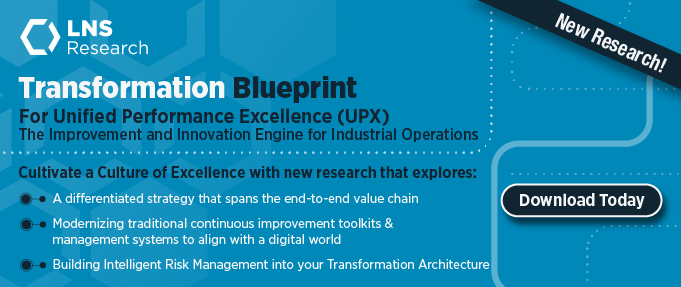