Advanced Industrial Analytics (AIA) is the common denominator of Industrial Transformation. You’ve heard this before from my LNS Research colleague Vivek Murugesan. AIA has applicability and added value no matter the focus of your transformation...especially quality.
For the uninitiated, we discuss the progression of analytics maturity in terms of the questions that can be answered, starting with descriptive analytics - which answers the question, “What happened?” We then progress to self-learning prognostic analytics, which answers the question, “What is the expected change in outcome given a prescribed action is taken?” (Figure 1)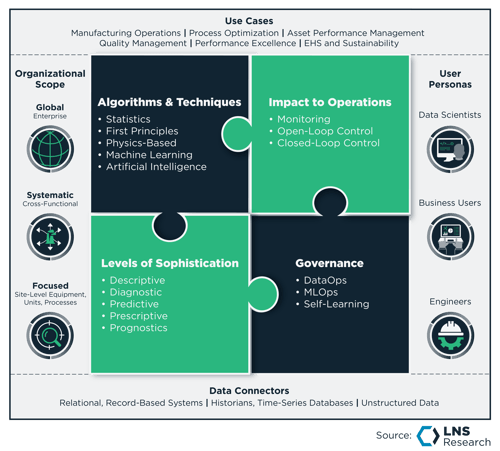
Figure 1: Advanced Industrial Analytics Capabilities
When we apply this lens to the analytics offered by many Enterprise Quality Management Software (EQMS) solutions, we often hear about predictive analytics. However, we frequently see Business Intelligence (BI) offerings primarily focusing on reporting against structured data within the system.
These representations of aggregated data provide the frequency of events or deviations in cycle time, allowing a VP of Quality to understand past audit effectiveness, time to close a CAPA, or recurring quality issues. However, within our analytics framework, these visualizations are squarely in the descriptive camp and are a far cry from enabling an enterprise to improve delivered quality outcomes proactively.
With few exceptions, this is what typically passes for analytics in EQMS.
How did we get here?
BI emerged in the 90s because enterprise applications struggled to analyze the structured data within the underlying database. Among many others, @Oracle (Hyperion) and SAP (Business Objects) made significant acquisitions. This didn’t just happen for ERP. In the mid-2000s, many MES vendors bought Enterprise Manufacturing Intelligence vendors simultaneously, and many EQMS vendors minted partnerships with BI vendors—Tableau and Spotfire being the two most prevalent.
Separately, for quality, statistical analysis-type BI products emerged. IBM bought SPSS, and SAS delivered its home-grown offering to the market. Both have quality and reliability statistical analysis tool sets that were broadly adopted – which in both cases were often grouped under the BI umbrella – even though they were slightly different but were in some ways closer to the Tableau and Spotfire type of BI that the EQMS vendors partnered with.
Even though an important step in the evolution, these acquisitions and architectures are not positioned to take advantage of today’s AI revolution. As semi-structured (time-series) and unstructured data grew, especially with the emergence of the cloud, traditional BI has been surpassed by modern data platforms offered by the likes of Snowflake, Databricks, and Palantir. As a result, data type-specific tool sets emerged, like PI vision, Seeq, and Trendminer for time series and the plethora of AI tools on top of unstructured data.
So, where does that leave us today? Momentum in the analytics technology market is with hyperscalers like AWS, MSFT, and Google, as well as the data platforms mentioned above. Forward-thinking and successful application providers like SAP and Siemens aren’t trying to compete in this space and, at this point, wouldn’t pay the multiples demanded by these types of companies anyway. So, they are partnering.
Forward-thinking EQMS vendors would benefit from forging these same types of partnerships, just as they did 10 or 20 years ago when BI vendors were ascendant.
What is the role of EQMS and Analytics in Delivered Quality?
There are several. I won't list them exhaustively, but here are the top five:
-
Prognostic product and process quality
-
Problem-solving and prevention
-
Uncovering relationships between variables for improvement
-
Predicting supplier performance
-
Predicting warranty and customer complaints
These five and most of the remaining analytics use cases cannot be performed exclusively in an EQMS or an Industrial Data Platform. This is why a systems-of-systems architectural approach is so critical.
When applications like EQMS interoperate with Data Platforms (Figure 2), data from the application can augment and improve what’s in the platform, and symbiotically, the data from the platform can augment and improve what’s in the application. Unfortunately, most EQMS today either do not have the needed connectivity capabilities or have not been architected to take advantage of the capabilities they do have.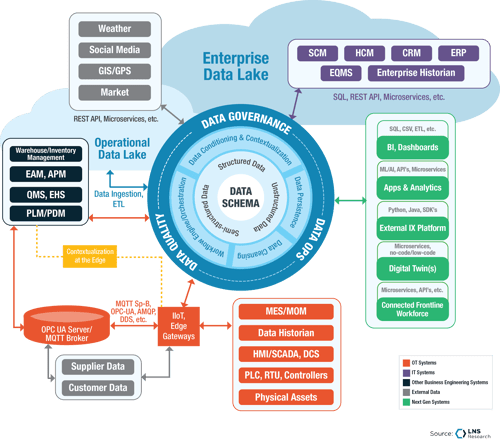
Figure 2: Data Platforms Make Data Accessible
For those ready to invest in this architecture, Industrial Data Platforms (IDP), Data Ops Software, and Industrial Applications Platforms (IAP) offerings have made significant progress over the past few years and excel at data ingestion, contextualization, and cleanliness. This set of capabilities makes clean, contextualized data available to Industrial Applications that sit on top, including EQMS. This approach enables a value-chain focus on Delivered Quality outcomes to customers, the north star of an Embedded Quality transformation.
Encouragingly, in our latest research on Embedded Quality, trust in insights derived from quality data is trending up, over 30% higher than in 2021, the last time we conducted this research. Embedded Quality Leaders are 154% more likely to trust analytics insights than followers and over 90% more likely to gain value from analytics insights. As demand and pressure for high-value analytics continue to grow, integration to the Quality Data Architecture layer will expand.
Recommendations for Chief Quality Officers:
-
Compliance is necessary but not sufficient. In most industrial companies, quality is a sub-function of a larger function that has some responsibility for delivering key parts of the company's value proposition. The quality function MUST pivot from compliance-focused activities to a focus on activating the value chain to deliver quality outcomes to customers first and compliance second.
-
Build on a Quality Data Architecture. Most EQMS scoping exercises start with a long list of required integration points. However, the reality of EQMS implementations is very different. Most required integrations are never implemented. EQMS is most often integrated with ERP and maybe an MES if one is in place. Integrations between enterprise applications are costly, time-consuming, and challenging to maintain. It is far easier, faster, and more efficient to enable a Quality Data Architecture that supports interoperability across the enterprise.
-
Lead by building a positive culture of quality. Quality professionals have a storied, credible history of driving data-driven decision-making, a much-needed skill set in today’s AI-crazed world. Quality professionals should focus on pushing the culture beyond compliance to using data and analytics to achieve a competitive advantage through delivered quality outcomes.
Recommendations for EQMS Vendors:
-
Move beyond structured data. Most EQMS today are document and event-driven platforms, and the data architecture is associated with those artifacts. In the LNS Research lexicon, we call that structured data. Artifacts generated outside of this context, things like pictures, audio, video, test, machine, and sensor data, are often unstructured. Advanced Industrial Analytics needs rich contextualized data from all these sources. EQMS Vendors should focus on enabling analytics for these other data types, focusing on critical predictive, prognostic, risk-based, and closed-loop quality use cases.
-
Make interoperability easy. The lack of EQMS integrations makes EQMS applications less “sticky” in the industrial enterprise. The less interoperable an EQMS is with other software systems, the easier it is to rip and replace, which happens in EQMS far more often than in other software, such as ERP, PLM, or MES. Establish a Quality Data Architecture with Industrial Data Platform players to expand reach across the enterprise exponentially and down to the shop floor to complete the last mile of quality.
-
Develop persona-first, use-case driven experiences. A seamless user experience is becoming table stakes. As workforce tenure and time in position tumbles precipitously, simplicity in interacting with operational software becomes essential to utility. The old days of having to log in and out of three or four different systems or applications to do one task are over. Ensure that the analytics you deploy through build, buy, or partnership are targeted at specific and prioritized user profiles, simplifying, automating, and augmenting decision-making processes.
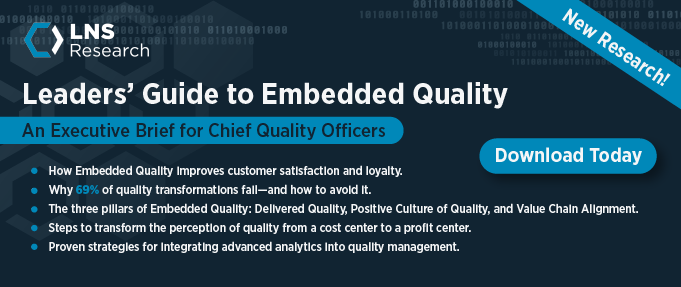