The Quality function in a typical industrial organization spans across multiple levels, from quality management, quality execution and control, research & development, laboratory inspection, supplier audits, customer warranty, etc. Not surprisingly, communicating between these functions requires consistent data and information transfers across multiple systems.
LNS Research's studies show that managing the quality data between these multiple systems is one of the biggest challenges faced by today’s Quality teams. In this blog post, we will describe some of the common ways Quality teams manage quality data, explain subsequent challenges, and provide recommendations for the Quality leader to maximize value from their quality data.
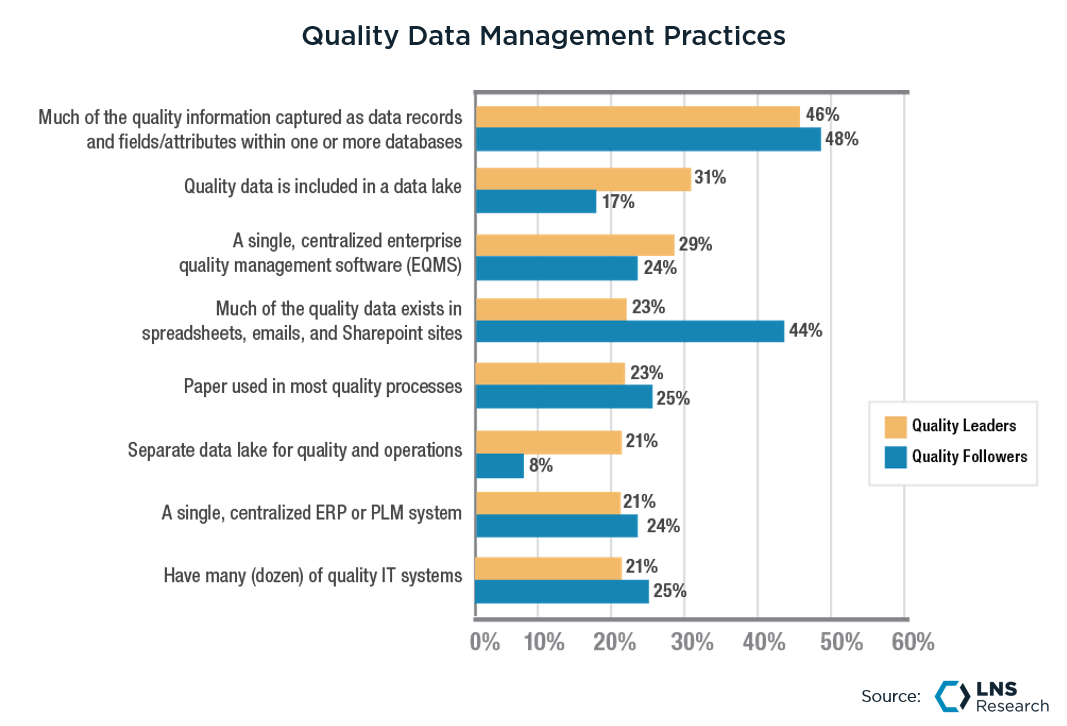
Challenge #1: Paper-based processes and systems are still prevalent in today’s plants
Paper-based processes in any business function are inefficient, inconsistent, unsecure, time-consuming, error-prone, and not analytics-friendly. This is a fact, and everyone in today’s industrial scenario knows this, and not surprisingly, digitizing paper-based processes is one of the top drivers for any transformation program.
However, as the above chart shows, almost a fourth of the surveyed companies claim to still use paper in their quality processes. The fact that even Quality 4.0 leaders - the companies that are ahead in Quality Transformation - cannot solve this problem proves how challenging it can be. The number one challenge in digitizing paper-based processes lies in the fact that it involves changing not just the data storage system but the underlying process.
Digitizing paper-based processes does not simply mean entering numbers into spreadsheets instead of writing them on paper. It requires companies to shed the don’t-fix-anything-that-works mindset, follow the “paper trail” up the value chain stream to the root cause, and digitally transform the entire process by either upgrading existing data collection mechanisms or investing in new ones.
Challenge #2: SharePoint and other flat-file systems that don’t provide the necessary depth
Apart from paper, the use of spreadsheets, word documents, PowerPoint files, and other flat file-based systems is also a common and sub-optimal way to manage Quality data. While this method overcomes several challenges in a paper-based process by enabling file sharing through email collaboration and SharePoint sites, it also causes its fair share of challenges.
These flat file storage systems may be capable of handling some parts of Quality data such as corrective and preventive actions (CAPA) data, documents storage, regulatory standards information, etc., but they are not equipped to drill down or provide additional information in case of audits or inspection. They also cannot support workflows necessary for automating Quality processes, making it tough for change management.
Challenge #3: Disparate IT and OT systems that don’t natively communicate with each other
The most common way today’s Quality organizations manage their Quality data is through a (complex) network of IT and relational database systems often managed by several teams. These systems include enterprise-level PLM, ERP, SCM, CRM systems, and plant-level MES, Data historians, SPC, LIMS systems, etc.
The majority of companies prefer using this assortment of systems for multiple reasons, including data security, familiarity with workflows and processes, and a deep-rooted resistance to change. However, these systems pose several shortcomings, such as integration, data accessibility, and connectivity restrictions.
Recommendations for the Quality Leader:
-
-
-
Invest in machine connectivity:
When it comes to Quality technology, almost every Quality leader has the word EQMS (Enterprise Quality Management Software) at the top of their transformation to-do lists, but they often overlook some necessary prerequisites before shopping for an EQMS. This involves steps like developing robust machine connectivity in the plant, implementing a common data model across IT and OT data, and creating data custodian roles (data engineers, data wranglers, etc.) to manage data quality and contextualize the data before it can be consumed.
-
EQMS systems address the majority of challenges in quality data management:
LNS Research believes that Enterprise Quality Management Software (EQMS) is the most efficient way to manage Quality data for most companies. Most EQMS systems are record-based, with several modules designed to effectively handle common Quality processes such as NC/CAPA, document management, supplier quality, regulatory standards, etc.
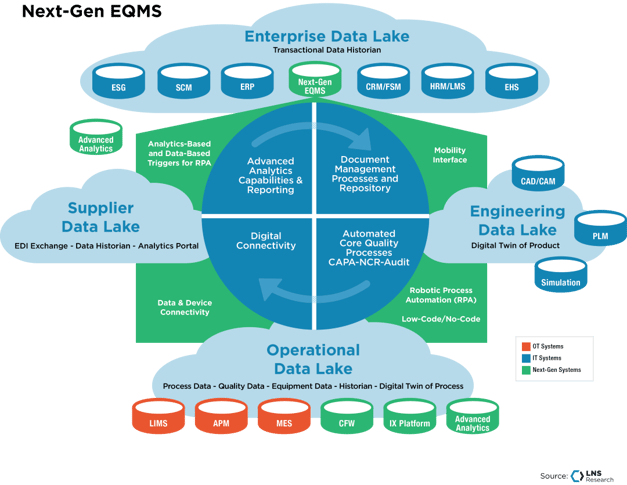
However, it is also one of the least common ways (2021 EQMS adoption rates stand at 28%). The reason why only a fraction of industrial companies have adopted an EQMS system is that it costs substantial amounts of time, money, and resources. While some of the leading EQMS vendors have developed cloud-native multi-tenant solutions that don’t need a lot of IT hours to implement, they still require end users to painstakingly disrupt, change, and transform Quality processes across multiple levels if customization is required.
-
Go Beyond EQMS systems with Data Lakes:
In addition to an EQMS, some of the early adopters have begun implementing data lakes to store Quality data. A data lake is a centralized data repository that can store multiple types of data in its raw format. Data lakes are mostly used for running advanced machine-learning algorithms on a large set of data by data scientists.
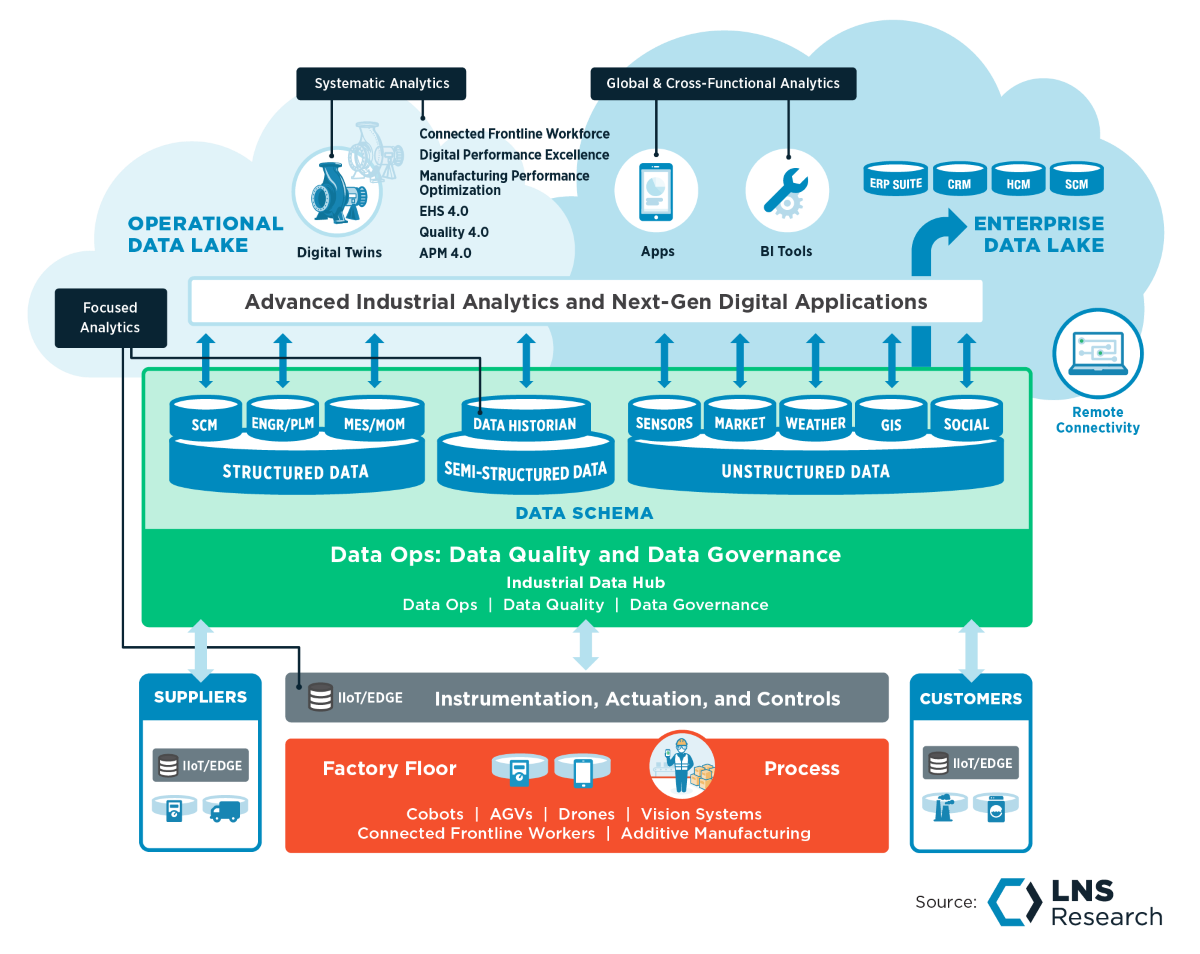
Quality teams could benefit from the data lake’s ability to perform ML/AI algorithms through analytics use cases such as sentiment analysis on social media consumer data, classification algorithms on supplier scorecards, multi-variate regression on process parameters, etc. However, without rigorous data governance and data ops practices, data retrieval and analytics from the data lake become more complicated, eventually turning the data lake into a data swamp.
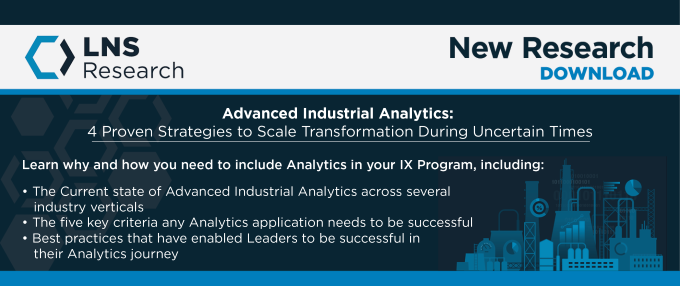
All entries in this Industrial Transformation blog represent the opinions of the authors based on their industry experience and their view of the information collected using the methods described in our Research Integrity. All product and company names are trademarks™ or registered® trademarks of their respective holders. Use of them does not imply any affiliation with or endorsement by them.