There’s a lot of buzz around Big Data these days. Sometimes it seems like we can’t view a tech blog or a Twitter feed without being bombarded by the term, ad nauseam. Whether you think it’s a current challenge or a bunch of hype about nothing, there’s one thing to keep in mind: while everyone’s drooling over Big Data, ‘Small Data’ might be getting lost.
That’s what Dan Somers believes, for example. He delivered a presentation on the subject at last month’s SAP Manufacturing Industry Forum near Chicago, IL., where LNS’s own Matthew Littlefield also spoke. I caught up with Somers after the event and learned more about his approach to what might be the next field in data analytics.
In this article we’ll look at an innovative approach to data and root-cause analysis, and discuss how Motorola used it to identify and correct a costly No Fault Found (NFF) return issue.
When Six Sigma Goes South
So, Six Sigma is a pretty rigorous model for process improvement and minimizing errors in manufacturing, right? That may be true, but sometimes results vary. That’s what got Professor Dariusz Ceglarek’s gears grinding. The then-Wisconsin-based manufacturing data expert looked at information associated with Six Sigma failures across manufacturing industries to determine why processes like Six Sigma were slow to resolve issues and had gaps (and costs) in production quality and yield.
Somers stumbled upon the professor’s work and the two found an intellectual synergy that resonated: They wanted to find failure markers that could explain why things went wrong, when they went wrong, and why they couldn’t be explained. So they founded Warwick Analytics.
“What everyone else has been doing until now has been measuring out-of-tolerance failures,” Somers explains. “Whenever something goes wrong or trends outside tolerances, people get alerted and take action. That’s fine, but it treats symptoms, not causes. When processes are within tolerance and failures still occur, we use ‘intolerance failure analysis.’”
A New Value in Dirty Data?
We often talk about ‘dirty data’ – information inputted into a system that doesn’t necessarily reflect actuality. Our capacity to control a process, non-conformance, or deviation, is only as good as the data itself and the accuracy of those entering it into a system. In conventional approaches to statistical analysis, the bottom line is, if ‘garbage’ goes in, garbage comes out.
This approach doesn’t use non-statistical algorithms and doesn’t need hypotheses or data that’s been cleaned of outliers or other ‘noise.’ Nor does it need to meet failure quotients to find statistical significance. The system always comes back with a set of business rules—a ‘fault region’—that are ‘correct.’ If the data are ‘dirty,’ the system still allows for corrective actions and identifies data to be gathered in the future.
It also deals with disparate, heterogeneous, low-level data coming from different sources: maintenance, warranty, testing, supply chain, and ERP, for example—the “boring stuff” as Somers calls it. And it can work with Big Data as well as limited datasets or ‘Small Data.’
Sounds fine in principle. But what about in actuality? For that, let’s go to the home of Six Sigma itself: Motorola, which invented the now-widespread process improvement framework back in 1986.
How Motorola Found the Needle Outside the Haystack
Like any company dealing with complex manufacturing, Motorola needed to solve a specific set of quality issues—in this case, on a particular mobile phone model. Some NFF audio and battery issues caused regular handset returns. While individual components tested well after being returned, it was clear some intermittent failure in the field was creating problems. NFF problems are challenging because it is difficult to recreate symptoms, let alone identify the causes behind the issues.
Motorola engineers and data specialists put their heads together and couldn’t find an explanation. So they turned to this unconventional approach.
Originally, phones were tested to see if they fell within tolerance across 170 parameters before they left the factory. They took this data and included failure markers (the ones that had developed NFF issues) to find ‘intolerance failures’—that is, the unique combination of variables which were acceptable individually but in combination created failure. In some cases, a variable was correlated to a variable coordinated to another variable that related to the root cause. This is stuff standard SPC analyses don’t tend to evaluate.
“A lot of times the needle isn’t in the haystack,” Somers explains. And in this case, that was true. However, the algorithms were able to mine data on test results to isolate fault regions so engineers could input resulting information back into Statistical Process Control tools to obviate regions and eliminate the problem. Soon, return rates plummeted, warranty costs dropped, and NFF incidences disappeared, basically because Motorola wasn’t letting faulty phones leave the factory.
The Kettle as a Health Monitoring Tool?
At its heart, this approach to root-cause analysis can be thought of as signal-based data mining that finds information in boring, low-level, seemingly innocuous data. Look at is this way: In an old-age residence, we have a ton of traditional health monitoring metrics to determine whether a senior is doing well.
But what about the kettle?
Whether or not the kettle is turned on in the morning during a resident’s day is a substantial indicator of the health and well-being of the resident, though it is outside all common definitions of standard health-monitoring indicators. The kettle activity is what we can think of as a signal that lies outside of conventional tolerance analysis. Instead it sits in the realm of ‘intolerance failure analysis’ framework, but speaks as directly to the ultimate results we look for.
This emerging field aligns well with where LNS Research believes data analysis could be going. As alluded at the beginning of this article, Big Data is often only as good as the ‘Small Data’ it is capable of capturing within all the noise its big brother presents. It will be interesting to see how companies like Warwick grow and attempt to help companies like Motorola achieve operational excellence by finding the markers between the lines to bring SPC to the next level.
We can only go so far in terms of user adoption, user acceptance, and establishing the veracity of data inputted into a system, but new models have the potential to circumvent these issues.
"We don't assume any data are wrong,” as Somers explains. “Everything has happened for a reason: if we get clusters, outliers, other data points close to that outlier—Small Data, as it were—we don't make any assumptions, we just let the data do the talking.”
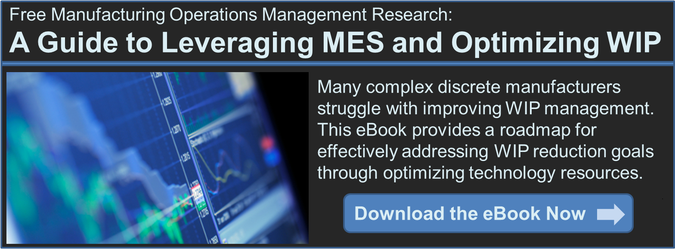