Over the past several months I have had the pleasure of attending many of the largest conferences covering the discrete and process manufacturing industries as well as working with many thought leading Big Data vendors. Without fail, two of the top issues discussed have been the rise in importance of the Industrial Internet of Things (IIoT) and the resulting implications for Big Data analytics in manufacturing.
In these discussion I have noticed two distinct viewpoints:
- “Manufacturing has always had Big Data. We have been collecting data with historians, MES, and EMI systems for decades. It is just a new buzz word for the marketers.”
- “Manufacturing is an untapped market for Big Data. There is lots of data, lots of different types of data, and hardly any of it is being used for analysis today.”
In considering these viewpoints, I would start by contending it is always useful to approach any new marketing term (or analyst framework) with a healthy dose of skepticism but, for myself, I fall in the second camp. I agree that we have always had “a lot of data” in manufacturing, but this is not what most industries have come to understand as “Big Data.”
It is not uncommon in manufacturing to hear of Smart Connected Assets like jet engines producing petabytes of data each flight or Manufacturing Execution Systems (MES) collecting millions of process variable measurements from the plant each shift; however, running reports on large data sets does not qualify as Big Data analytics in manufacturing.
Defining Big Data Analytics in Manufacturing
So if Big Data Analytics in manufacturing is about more than the amount of data, how should we as an industry define Big Data analytics in manufacturing? At LNS Research, we define Big Data analytics in manufacturing the following way:
Big Data Analytics in manufacturing is about using a common data model to combine structured business system data like inventory transactions and financial transactions with structured operational system data like alarms, process parameters, and quality events, with unstructured internal and external data like customer, supplier, Web, and machine data to uncover new insights through advanced analytical tools.
This definition of Big Data Analytics differs from the traditional approach most manufacturers and vendors have taken to dealing with manufacturing data. In most cases, manufacturers have invested heavily in data collection and visibility, mainly through legacy MES, EMI, and Data Historians.
In particular, EMI has largely been understood as a two-fold integration and dashboard tool where many vendors have invested heavily in both proprietary and open integration with ERP and Automation systems as well as in dashboard and mobile technologies to bring metrics to decision makers when and where they need the right information.
Therefore, EMI offerings today need to transform in three distinct ways to be truly considered Big Data Analytics in Manufacturing
- Move out of the MOM space to be able to move data from anywhere to anywhere with open technologies
- Invest in a data model that can handle structured and unstructured data from anywhere in the system architecture stack inside or outside the four walls of the factory
- Apply new analytical tools to this new data model to enable never before possible insights. Examples of these analytical tools would be: Image, Video, Geospatial, Time Series, Predictive Modeling, Machine Learning, Optimization, Simulation, and Statistical Process Control.
Implications for Traditional Big Data and Start-Ups
Of course the existing EMI vendors are not the only players in the space that want to play in Big Data analytics in manufacturing; there are also a number of exciting startups as well as the legacy BI vendors.
For most of these companies the starting point for any Big Data analytics solution has been IT and enterprise systems. This has both pros and cons. By coming from an IT background, these providers have an understanding of structured and unstructured data and the analytical tools needed to deal with this variety in data types. However, on the flipside, most of these vendors have not dealt with the type of real-time data found in manufacturing, and have also not dealt with the resource constraints manufacturing faces.
Namely, manufacturing organizations do not have the data scientist and often don’t even have business analysts many times found in IT departments and are needed to provide the time and effort for refining data models, massaging analytical tools, and teasing out insight iteratively over the course of weeks and months.
Instead, manufacturers have process experts, operational excellence teams, and engineers. These individuals are smart and capable with an intimate understanding of the manufacturing process, but need simple and intuitive analytical tools to pull the value out of data. These individuals are craving much more than a simple dashboard but also don’t have the time or expertise to be dealing with statistical programming languages like R, SAS, and SPSS to be designing and configuring the next new algorithm to predictively model their process.
The IIoT Platform and Next-Gen Applications
As the space continues to mature, it is likely that Big Data Analytics for manufacturing will become part of the IIoT Platform for delivering both legacy applications and Next-Gen systems.
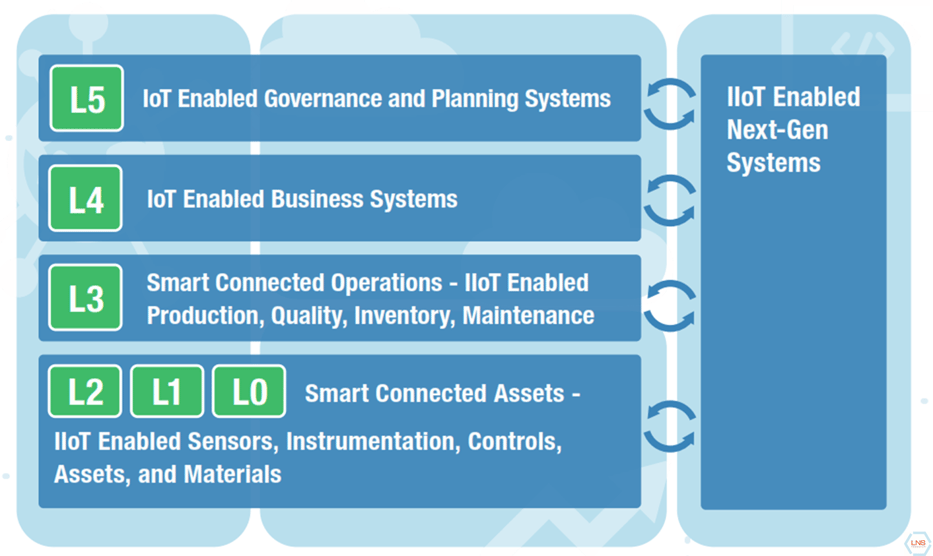
It's the Next-Gen systems that will make up the new IIoT Application Workspace. These will be the applications that can fill in the white space from traditional architectures and will take data from anywhere and deliver it to anywhere else for new analytics and new mashup applications. These will also be the applications that simplify the analytics to be useable for shop floor personnel and/or couple these solutions with the necessary services and data scientist expertise.
Join me tomorrow in a free webinar as I dive deeper into the current state of the IIoT, where companies and industries are within their awareness and investments, and what's needed to push this revolutionary space forward.
Gain a year of free access to new research in our IoT Research Library by completing a survey.