The IoT offers exceptional promise in the field of Asset Performance Management (APM). Some of the first true benefit use cases are emerging in APM, using data from the IoT in manufacturing plants with advanced analytics to better predict failures of production machinery, which leads to service prior to unexpected downtime.
At the same time, the ever-increasing use of automation and process control, including advanced forms of control like adaptive control, is leading to higher productivity levels and much greater per person efficiencies in our manufacturing plants.
The press is full of stories touting the great things the IoT will deliver in the future and promoting automation to improve productivity and quality. Yet, there is a downside to everything, and the unintended consequences of automation often appear in the form of impacts on asset life and performance.
Before the Age of the Smart Machines
In the “good old days” when automation was predominantly single-loop control or simple relay logic-based, automation operators established a process setpoint, and then manually adjusted that setpoint to maintain the balance of throughput and quality.
As process degradation occurred due to normal wear and tear of the manufacturing equipment, it became harder and harder to maintain the process “on-target” with either quality or throughput starting to vary beyond the ability of the operator to adjust the setpoint to compensate.
At that point, the operator would call for maintenance assistance to “fix” the equipment so that manufacturing targets could be maintained. Often this entailed merely making an adjustment or some minor mechanical repair with more extensive service scheduled for the next period of normal downtime.
Sometimes Automation Is Too Good
With the advent of digital computing, along came much smarter control and automation systems capable of far more sophisticated control. With the power of the computer, a control system could make process parameter adjustments in real time to accommodate virtually any degree of variation in the process. So as equipment becomes ever-more unstable due to wear-out, the control system just works harder and is still able to maintain production throughput and quality targets.
Since production objectives are being met, and the control system--unlike its human counterpart--never complains about being asked to work too hard, process equipment can reach a point of catastrophic failure before anyone notices. Now instead of a minor adjustment or service, when trouble occurs it can cause significant downtime and be extremely costly both in terms of parts and labor.
Manufacturing’s approach to alleviating this problem has been to implement first preventative maintenance (PM) based on calendar time, then basing the PM on actual usage, either run-time or cycle counts. The next stage was to move to predictive maintenance (PdM), based on derivative indicators such as operating temperatures, vibration, or lubricant contamination or degradation, as appropriate.
With more and more derivative data being made available via the IoT, we can--through the use of advanced analytics--more accurately predict pending issues and avoid those costly catastrophic failures.
Don’t Overlook the Obvious
So with the IoT, big data analytics, and ever-increasingly sophisticated knowledge about process equipment condition, we are now able to reduce the downtime that our adoption of technology led to in the first place. This isn’t to condemn automation in technology, as it clearly has improved productivity, quality and the ability of manufacturers to compete in a global economy where cheaper labor is always just a country away. Instead, this is a call to not overlook the obvious when setting up your APM PdM programs.
Looking back, the trigger for maintenance when humans were a far more integral part of the process was simply that the operator had to expend so much effort trying to control the process that they called for maintenance support. Yet, today, we rarely measure the control effort the automation system expends in doing the same thing. Not that we shouldn't measure derivative factors like equipment condition based on temperature, vibration, or energy usage, but I argue that if we just looked at how hard the controller is working to maintain control, provided we have a stable raw material supply, we can make more informed decisions about the process stability and hence the state of the physical assets.
This serves as a call to all of the automation vendors to consider this as they build out their APM portfolios going forward.
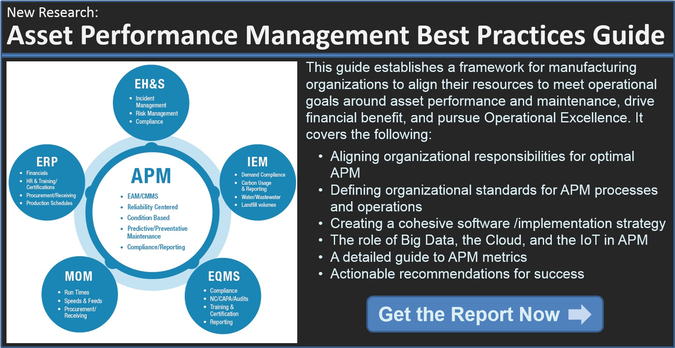