Advanced Industrial Analytics programs are at a pivotal stage in today’s manufacturing industry. LNS’ latest Analytics That Matter research shows that a third of recently surveyed industrial companies have fully engaged in Advanced Analytics programs, and almost twice as many are running pilots or have budgeted to do so within the next 12 months.
In terms of returns, while a handful of these companies have already begun realizing dramatic step-change benefits from these programs, the remaining majority are still stuck with minimal or stagnant results, facing a variety of challenges.
In this blog post, we will be briefly discussing the top three challenges faced by industrial companies in their Analytics journey- data quality, legacy systems, and gathering buy-in from the plant.
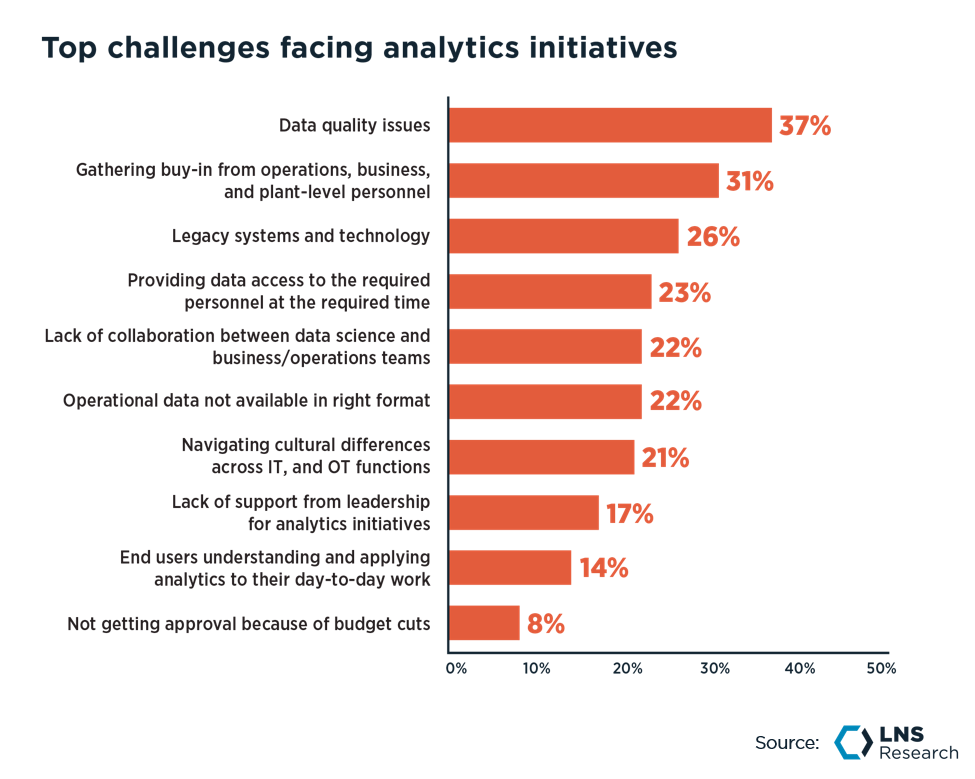
Data quality issues
As shown in the above chart, data quality is the number one challenge facing Analytics initiatives in manufacturing companies. Not surprising, given that I’ve heard multiple accounts of analytics and data science teams spending as much as 70-80% of their time and efforts on data preparation and cleaning as opposed to analysis. While it is important to perform data cleaning, it is even more important to know where to do it and where to leave the data as is.
Some of the common data quality issues include missing data, incorrect data, anomalies, data not being in the required format, data without context, etc. Interestingly, while most companies have the required skill set, and even the right technology in some cases to manage these data quality issues, what they usually lack is a set of standardized governance processes to enforce them.
As a result, some of the leading companies have adopted DataOps, which combines Agile, DevOps, and Lean methodologies to ensure high levels of data quality. For instance, some of the common DataOps practices include creating data custodian roles at multiple levels in the organization, pushing data quality responsibilities as close to the source as possible, etc.
There has also been significant activity in data quality on the vendor landscape. Several of the major Industrial Analytics vendors have recently included some data quality and conditioning & contextualization functionalities as part of their offerings, and a few new ones have positioned themselves as DataOps, Data Quality, and Reliability solutions.
Legacy systems and their data models
Apart from data quality, a major challenge facing Analytics is legacy systems. These are the older generational enterprise systems or databases that burden almost every single company with limited functionalities, outdated UI, and minimal interoperability. Despite these flaws, these systems cannot be ripped and replaced as they are too integral to existing workflows and the underlying architecture.
Connecting data from and to these legacy systems takes up substantial amounts of time and resources for most Analytics programs today. In attempting to solve this problem, many companies have tried to bring existing legacy systems and other data sources together into a common data model. However, since each of these systems has its own data models and structures, it will be an extremely difficult and even futile task to build custom integrations and APIs to do so.
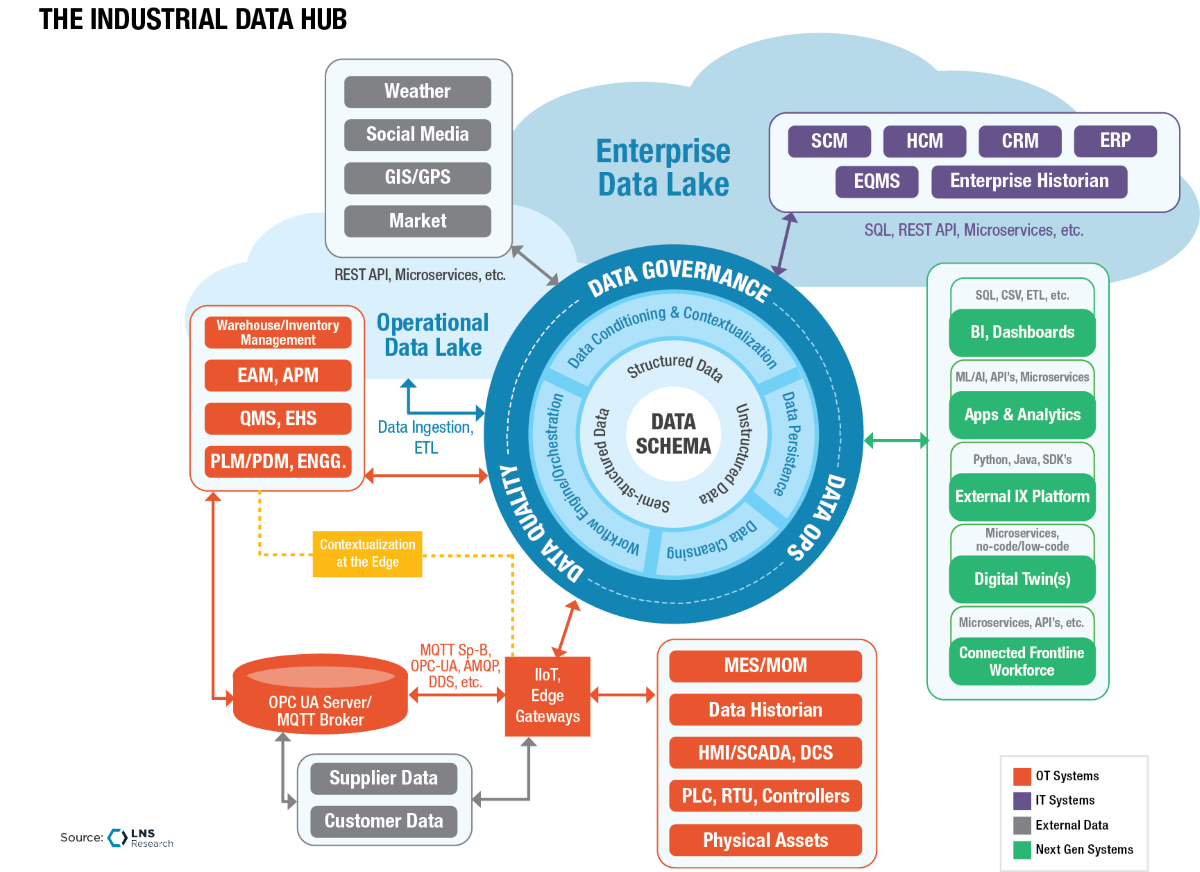
On the other hand, a more efficient strategy could be to build a graph-based data schema, which can be thought of as something like a digital thread that maps common attributes and elements (nodes) across these data sources. This incremental data schema, along with an agile approach can help overcome a lot of the traditional data mapping challenges associated with building a common data model that usually takes several years.
The above-shown Industrial Data Hub is LNS Research's representation of how the data schema could map existing legacy applications with some of the modern platforms and applications.
Gathering operations’ buy-in
Apart from data and technology, there are significant organizational challenges to analytics as well. This third type of challenge mostly arises from the fact that Analytics initiatives are often driven by IT or digital leaders, without the involvement of plant-level engineers, subject matter experts, and the frontline workforce.
Because of this, many plant-level teams don’t end up actively partaking in any Analytics initiatives and prefer their paper-based and gut-backed decisions to do their job. Additionally, there is a job-security element as well. A major reason why so many frontline workers don’t prefer modern data-driven processes is that they are wary that they would replace their jobs eventually.
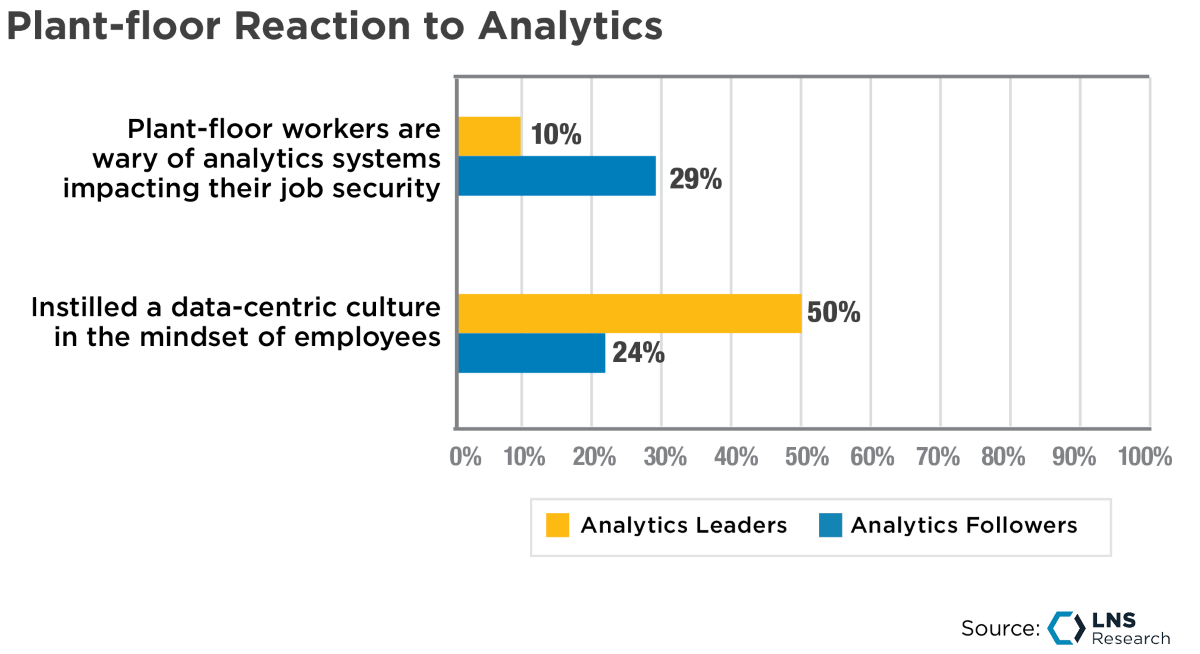
The above data points show that this is a common challenge among almost a third of the followers. On the other hand, the leaders have managed this challenge by instilling a data-centric culture in the mindset of employees. This data-centric culture democratizes the use of data across the organization (not just IT and digital teams), ensuring that the operations and plant-level personnel:
-
-
-
have the necessary access to the required data in the required format,
-
trust the data,
-
and can make timely decisions based on it.
Nurturing this data-centric culture and involving the plant-level personnel in the Analytics journey ensures that they support and even act as champions of these Analytics initiatives.
Summary & Recommendations
Today’s manufacturers are at a critical point in Analytics. While the industry leaders have already laid the foundation and are now accumulating step-change benefits, the remaining majority are lagging and must move fast and quickly address challenges to be able to catch up.
The challenges mentioned in this post are in no way an exhaustive list; this was just a subset of some of the more common ones faced by manufacturers. LNS Research recommends companies struggling with their Analytics programs start addressing these challenges by:
-
-
-
Making data quality - the top challenge facing Industrial Analytics programs - a priority. Data quality is not as simple as ensuring the data is available to the required teams, but also making sure it’s in the required format, has the required context (meta-data), and is available in the required time. More on data quality is to come in our next research spotlight of the Analytics That Matter series.
-
Instilling and nurturing a data-centric culture by democratizing the use of data across the enterprise. This ensures that the plant-level engineers, managers, subject matter experts, and operators are bought into the vision set by leadership.
-
Implementing DataOps and governance processes to ensure high levels of data quality and context. This includes creating teams, forming cross-functional councils, and even executive-level leaders with the right tools and responsibilities to treat data as an asset.
-
Not spending substantial time and effort in trying to build a holistic data model, but rather taking a more agile and incremental approach to building a graph-based data schema by mapping common elements and attributes.
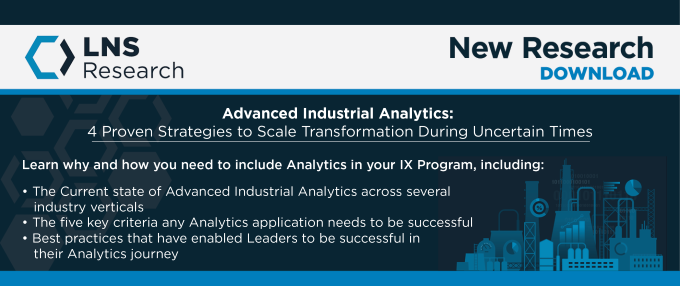
All entries in this Industrial Transformation blog represent the opinions of the authors based on their industry experience and their view of the information collected using the methods described in our Research Integrity. All product and company names are trademarks™ or registered® trademarks of their respective holders. Use of them does not imply any affiliation with or endorsement by them.